Meta’s research on motion tracking for AR/VR applications using wearable sensors addresses the need for realistic pose replication. By combining headset and controller poses with physics simulation and environment observations, the company achieved accurate full-body poses in constrained environments. Meta’s physics simulation automatically enforces pose constraints, resulting in high-quality interactions. Key features like environment representation, contact reward, and scene randomization are discussed. While praised, some researchers highlight the ongoing issue of restricted access to sensor data for developers, which may limit customization and hinder development opportunities. Granting users control over their data is crucial for advancing XR technologies.
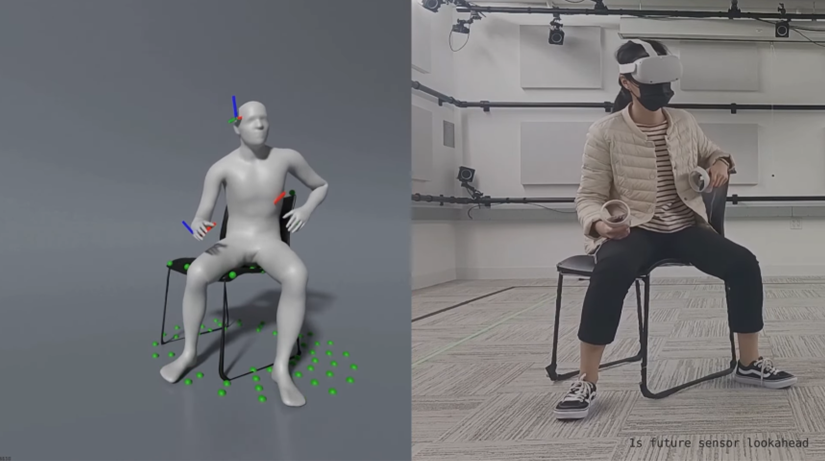
For many AR/VR applications, replicating a user’s pose solely based on wearable sensors is crucial. However, most existing motion tracking methods only consider foot-floor contact and neglect other environment interactions due to their complex dynamics and strict constraints. In daily life, people frequently interact with their surroundings, like sitting on a couch or leaning on a desk. By employing reinforcement learning, Meta demonstrated that combining headset and controller poses with physics simulation and environment observations can generate realistic full-body poses even in highly constrained environments.
The physics simulation, says Meta, automatically incorporates the necessary constraints for realistic poses, eliminating the need for manual specification common in kinematic approaches. This approach ensures high-quality interaction motions without typical issues like penetration or contact sliding. In its research paper, Meta discusses three essential features that significantly contribute to the method’s performance: environment representation, contact reward, and scene randomization.
To showcase the method’s versatility, Meta provided examples of various interactions, such as sitting on chairs, couches, and boxes, stepping over boxes, rocking a chair, and turning an office chair. We believe these results represent some of the highest-quality outcomes achieved for motion tracking using sparse sensors with scene interaction.
Although the study is highly praised, some researchers point out that it highlights a persisting issue with many devices: the lack of access to sensor data for developers to utilize their own computer vision (CV) methods.
This could result in this tracking feature becoming a prepackaged functionality with limited customization options and restricted access to the underlying data. That is reminiscent of the situation with hand tracking, which is an impressive advancement but also an illustration of policies that hinder broader development opportunities.
Users should have the ability to control their own data, suggest researchers outside of Meta. If they choose to share their camera imagery with an application, they should be permitted to do so. The current restrictions are holding back the progress of extended reality (XR) technologies.